
Artificial Intelligence in Drug Discovery and Development
The use of Artificial Intelligence (AI) in Medicinal Chemistry has gained significant attention in recent years as a potential means of revolutionizing the Pharmaceutical industry. Drug discovery, the process of identifying and developing new medications, is a complex and time-consuming endeavor that traditionally relies on labor-intensive techniques, such as trial-and-error experimentation and high-throughput screening. However, AI techniques such as Machine Learning (ML) and Natural Language Processing offer the potential to accelerate and improve this process by enabling more efficient and accurate analysis of large amounts of data. AI-based methods have also been able to predict the toxicity of drug candidates. These and other research efforts have highlighted the capacity of AI to improve the efficiency and effectiveness of drug discovery processes. However, the use of AI in developing new bioactive compounds is not without challenges and limitations. Ethical considerations must be taken into account, and further research is needed to fully understand the advantages and limitations of AI. In spite of these challenges, AI is expected to significantly contribute to the development of new medications and therapies in the next few years.
Generative AI in Pharma Industry
Generative AI is a type of Artificial Intelligence (AI) that can create new content, by learning from existing data to create new data that is similar in style and content. Generative AI is a rapidly developing technology that has the potential to revolutionize many industries, including the pharmaceutical industry. Generative AI can be used to create new drugs, improve the efficiency of drug discovery, and personalize treatments for patients.
One of the most promising applications of generative AI in the pharmaceutical industry is the development of new drugs. Generative AI can be used to create new chemical compounds that have the potential to be more effective and less harmful than existing drugs. Generative AI can also be used to design new drug delivery systems that can improve the efficacy and safety of drugs.
Generative AI can also be used to improve the efficiency of drug discovery. It can be used to screen large databases of chemical compounds to identify potential drug candidates and to design experiments and analyze data. This can help pharmaceutical companies to discover new drugs more quickly and efficiently.
Finally, generative AI can be used to personalize treatments for patients, create models of the human genome and predict how patients will respond to different drugs. This information can be used to develop personalized treatment plans that are more effective and less harmful than traditional treatments.
The Role of Machine Learning (ML) in Predicting Drug Efficacy and Toxicity
One of the key applications of AI in medicinal chemistry is the prediction of the efficacy and toxicity of potential drug compounds. Classical protocols of drug discovery often rely on labor-intensive and time-consuming experimentation to assess the potential effects of a compound on the human body. This can be a slow and costly process, and the results are often uncertain and subject to a high degree of variability. AI techniques such as ML are able to overcome these limitations. Based on the analysis of a large amount of information, ML algorithms can identify patterns and trends that may not be apparent to human researchers. This can enable the proposal of new bioactive compounds with minimum side effects in a much faster process than when using classical protocols. For instance, a DL algorithm has recently been trained using a dataset of known drug compounds, along with their corresponding biological activity. The algorithm was then able to predict the activity of novel compounds with high accuracy. Significant contributions to prevent the toxicity of potential drug compounds, employing intensive training using large databases of known toxic and non-toxic compounds for ML, have also been published.
Another important application of AI in drug discovery is the identification of drug–drug interactions that take place when several drugs are combined for the same or different diseases in the same patient, resulting in altered effects or adverse reactions. This issue can be identified by AI-based approaches by analyzing large datasets of known drug interactions and recognizing the patterns and trends. This has been recently addressed by an ML algorithm used to accurately predict the interactions of novel drug pairs. The role of AI to identify possible drug–drug interactions in the context of personalized medicine is also relevant, enabling the development of custom-made treatment plans that minimize the risk of adverse reactions. Personalized medicine aims to tailor treatment to the individual characteristics of each patient, including their genetic profile and response to medications.
Advantages & Disadvantages of Artificial Intelligence in Drug Discovery
Advantages
- Eliminates Human Error and Risk
- 24/7 Availability
- Unbiased Decision Making
- Repetitive Jobs
- Cost Reduction
- Data Acquisition and Analysis
Disadvantages
- Costly Implementation
- Lack of Emotion and Creativity
- Degradation
- No Improvement with Experience
- Reduced Jobs for Humans
- Ethical Problems
Don’t miss out! Click here to stay in touch.
Categories
- Biopharma (53)
- Consumer Health (15)
- Cosmetics (8)
- Diagnostics (6)
- Digital Health (6)
- Food (1)
- Medical Device (98)
- OTC (3)
- Regulatory Intelligence (5)
- Standards (34)
Recent Blogs
Get the latest updates from Vistaar
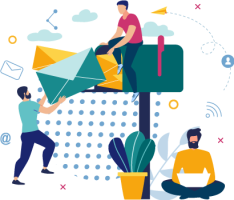
Related Posts
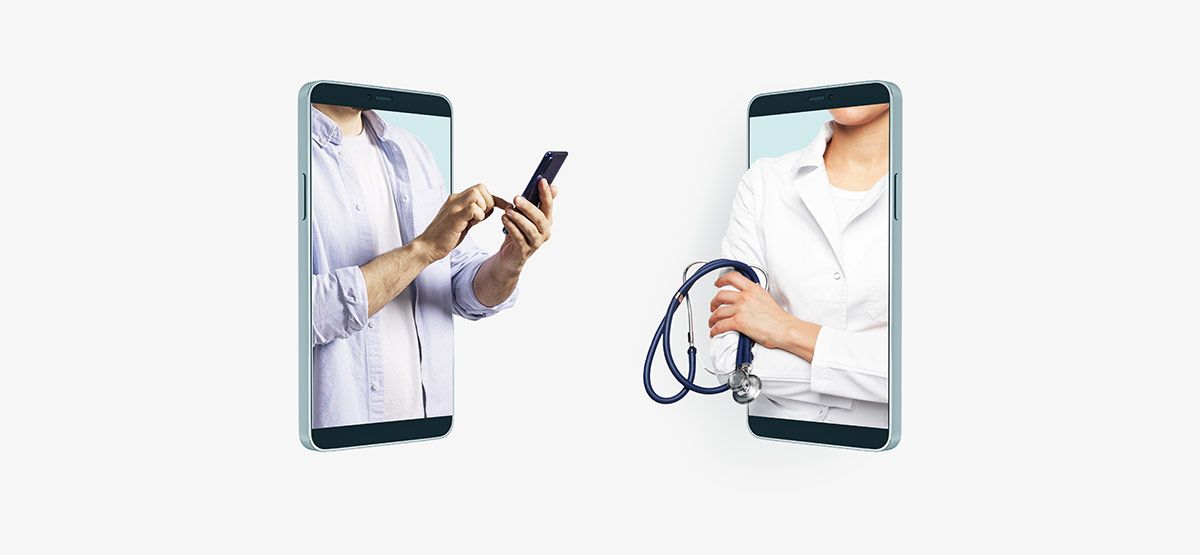
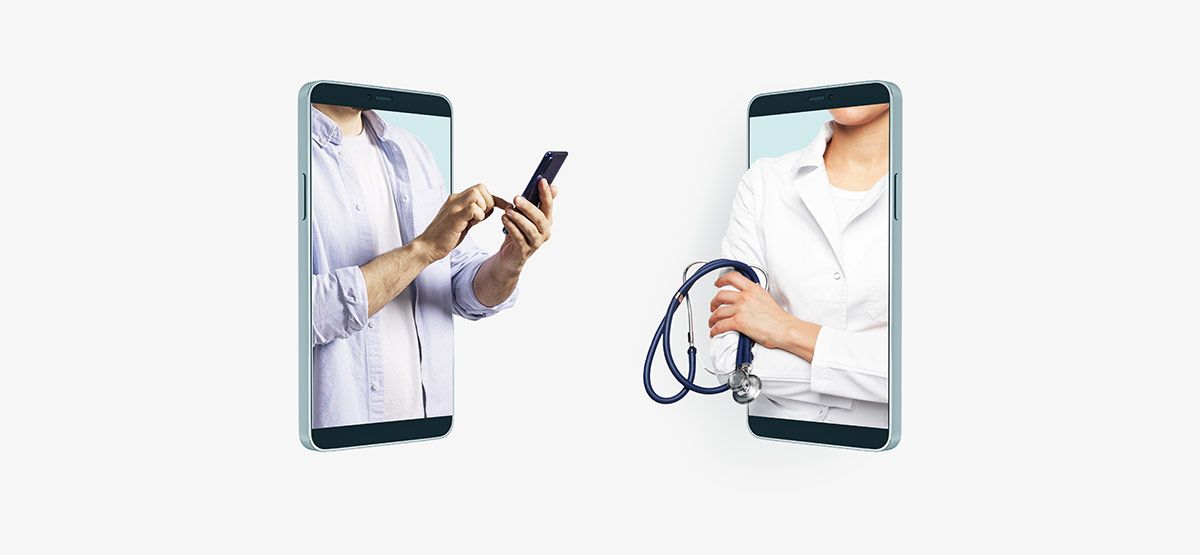
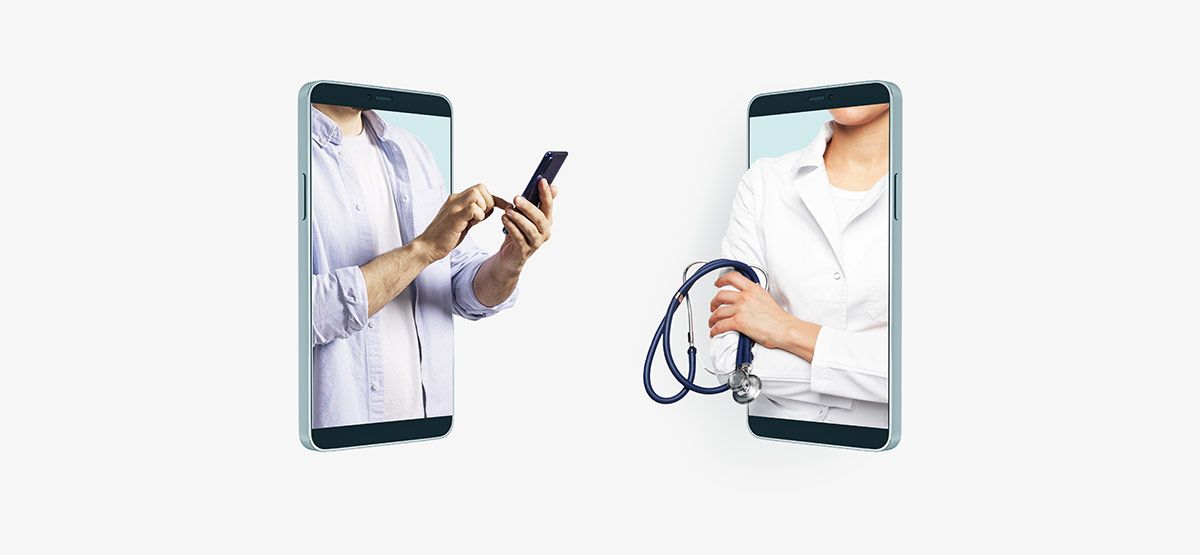
The Role of Regulatory Tracking Software in Accelerating Drug Approval Processes
CONNECT WITH US
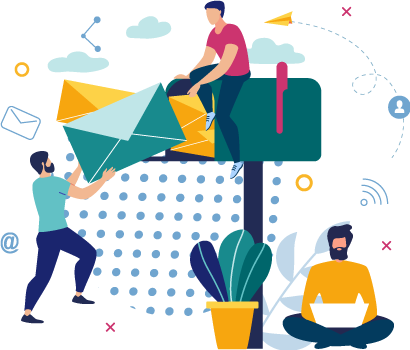